Stray Field NMR for Osteoporosis
Osteoporosis is a disease in which the density and quality of bone are reduced, affecting mainly post-menopausal women.
For now, there is no easy method to monitor early changes in BMD (bone mineral density) to assess hormonal treatment efficacy in osteoporotic patients, as existing tools are not sensitive enough. The goal of our project is to develop a small, simple, affordable, MR-based table-top scanner to detect cellular changes in fat percentage in bone marrow as an early indicator of treatment efficacy.
The Scientific Basis: Bone marrow adiposity in postmenopausal women increases with osteoporosis. Hormonal changes associated with the postmenopausal phase trigger the pathways that alter the differentiation of mesenchymal stem cells into adipocytes, disrupting the equilibrium between adipocyte and osteoblast differentiation. This process starts long before BMD changes are obvious.
Experiments performed in our group demonstrated that changes occurring in the bone marrow that are related to the onset of osteoporosis and to its treatment, can be detected by the use of a mobile stray field NMR (1,2).
Based on these findings, we are developing a dedicated mobile stray-field NMR device that will allow monitoring bone marrow adiposity (Fig. 1).
References
-
Detection of Bone Marrow Changes Related to Estrogen Withdrawal in Rats with a Tabletop Stray-Field NMR Scanner. Sarda et al, Magnetic Resonance in medicine 2016.
-
Monitoring of Cellular Changes in the BoneMarrow following PTH(1-34) Treatment of OVX Rats Using a Portable Stray-Field NMR Scanner. Hillel et al, Journal of Osteoporosis, 2017.

Fig. 1. T1, ADC, and T1 measurements for bones of ovariectomized (OVX) rats and control from time of operation showing: (a) Statistically significant reduction in T1 relaxation time for the OVX rats at two time
points: 3.5 and 4.5 months. (b) Statistically significant reduction in ADC for the OVX rats at two time points: 3.5 and 4.5 months. (c) Statistically significant increase in T2 relaxation time for the OVX rats at two time points: 3.5 and 4.5 months.
Magnetic Exchange
Magnetic resonance imaging (MRI) is mostly performed on protons associated with freely moving water molecules, as they are highly abundant in biological tissues yielding high signals. Nonetheless, much signal information originates from the non-aqueous species in the tissue, such as proteins and lipids. We aim to implement a novel MRI technique that allows the acquisition of signal generated by the protons adjacent to the semi-solid components of the tissue and combine it with quantitation analysis to extract the volumetric fraction of proteins or lipids. The proposed MRI sequence, MEX (magnetization exchange), can provide an insight into the content of species with restricted motion. The MEX sequence is illustrated in Figure 1 below.
In the MEX preparation block a selective excitation is employed, yielding complete water suppression. The time interval tLM precedes the imaging module, in which magnetization recovery occurs via the two process: MT from the non-aqueous species to the free water and inherent water T1. This experiment is repeated with variations in the delay time (tLM) to acquire the full kinetics of the recovers signal and its dependence on the fraction of the motion-restricted protons.
The governing equation is equation 1 bellow
This new sequence in implemented on common mice model for Multiple sclerosis (MS) – Cuoprizone. Cuprizone, when fed to young adult mice, onsets demyelination process in lesion sites in the brain. The damage caused by the Cuprizone can be detected in the cellular, molecular and biochemical levels. Figure 2 depicts parameter maps and segmentation, for one Cuprizone fed and one control mice. It can be seen that the area of the CC in the Cuprizone mouse has lower values of F than the control animal, meaning a lower relative percentage of the myelin in this region. Also visible is an increase in T1 values for the Cuprizone fed animals, as to be expected in a less myelinated tissue.
Figure 3 displays the three fitted parameters in both groups. F is reduced by 25% (P<0.05), is lower by 59% (P<0.01) and T1 is higher by 37% (P<0.01). The expected effect of the Cuprizone model on myelin in the CC support these results of the F values36. In Figure 4, the F values, as fitted to the signal obtained from the Internal Capsule and grey matter (marked in Figure 2. a,e), are shown. In these results we cannot detect any significant decrease in F values, supporting the influence of Cuprizone administration mainly in the Corpus Callosum.
In order to compare them MEX sequence and analysis with the gold standard of myelin evaluation, histopathologic images were taken and examined. Figure 5 depicts the correlation between the histology analysis and the F values of all the animals, in both areas of white matter (CC) and gray matter.

Equation 1- governing MEX sequence equation

Figure 1 - MEX pulse sequence. Two selective pulses are applied at the water resosnance, each followed by a crusher. The delay time, tLM, between the preparation and the imaging module, was varied between repetitions.
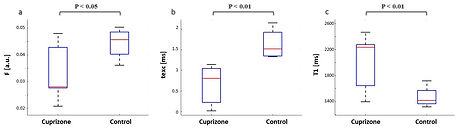

Figure 2 - Analysis results for two exemplary animals. The parameters are displayed as color-maps overlaid reference image in gray. Top row is cuprizone fed mouse and bottom is control mouse. Panels (a),(e) are the ROI's markings on reference image (FLASH): red – CC; green – GM; blue – IC. Panels (b-d),(f-h) are the fitted parameters, F, and T1, for each voxel with R2>0.98. White arrows in panel (f) indicate the enhanced F values in the CC.

Figure 3 - F, τexc and T1 parametrs for all cuprizone and control mice in Corpus Callosum. The central mark (red) indicates the median, and the bottom and top edges of the box indicate the 25th and 75th percentiles, respectively. The whiskers extend to the most extreme data points not considered outliers. (a) F values in cuprizone group is lower by ~25% (P<0.05); (b) texc values are lower in cuprizone compared to control by ~ 59% (P<0.01); (c) T1 values are higher in treated group by ~37% (P<0.01).
Figure 4 – F values in Cuprizone and control mice. (a) In the Internal Capsule and (b) in the Cortical Gary Matter. There is no significant difference in both areas.
Figure 5 – Correlation analysis between histological staining and F value extracted in white matter and gray matter areas in all animals
Brain Tumor response assessment
Volumetric measurements of brain tumors are important for accurate diagnosis and follow-up. However in clinical setup manual segmentation of high grade gliomas (HGG) is usually inapplicable as it is highly challenging, time consuming and user dependent. The aim of this study was to implement a deep learning (DL) approach to automatic longitudinal assessment of brain tumor response based on the clinical response assessment in neuro-oncology (RANO) criteria.
135 MRI scans obtained from 67 patients with HGG were included. All scans includes FLAIR, pre and post T1-weighted and T2-weighted images, as well as manual segmentation of lesion area into three components: enhancing, non-enhancing and necrosis.
A neural network with a U-net architecture was trained for identification and sub-segmentation of lesion components. Evaluation of the segmentation results was based on measurement of similarity between the components' contours based on dice similarity coefficient (DSC) score, mean accuracy, sensitivity and specificity, and consistency with the clinical RANO criteria
The mean results for the entire lesion area were: DSC = 0.88±0.06, sensitivity = 0.87±0.007 and specificity = 0.997±0.001. Based on RANO criteria, consistency in patient assessment in terms of complete or partial response, stable or progressive disease was obtained in 44/51 cases.

Figure 1: Segmentation results obtained from four patients with glioblastoma. (a) Post contrast T1WI (T1WI+C) (b) FLAIR images, and (c) segmentation results: red=enhancing component, blue=non-enhancing component, green=necrotic component
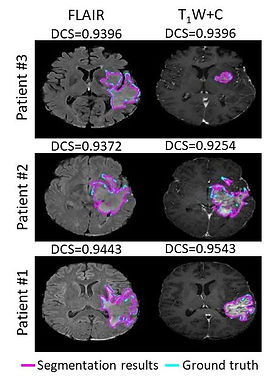
Figure 2: Examples of segmentation results obtained in three patients with glioblastoma, demonstrating the high similarity and DCS values obtained between the segmentation (magenta) and results ground truth (light blue).

Figure 3: Longitudinal segmentation results obtain in a 62 year old male with 4 scans, once every ~2 months, showing the high similarity between the DL segmentation results (dashed line) and the ground truth (solid line) for all lesion components (red=enhancing component, blue=non-enhancing component, green=necrotic component).